About RainDrop's Data
Learn more about how RainDrop derives its rainfall estimations and the challenges it faces.
Posted by
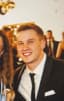
Related reading
Total Precipitation Averages For June
Discover how much precipiation falls on average in June! This map shows Junes's precipitation totals for the United States.
Total Precipitation Averages For May
Discover how much precipiation falls on average in May! This map shows May's precipitation totals for the United States.
Best Rain Gauge App For Farmers in 2024
Learn why RainDrop is the best rain gauge app for farmers seeking rainfall measurements for each of their fields without the hassle of physical gauges.
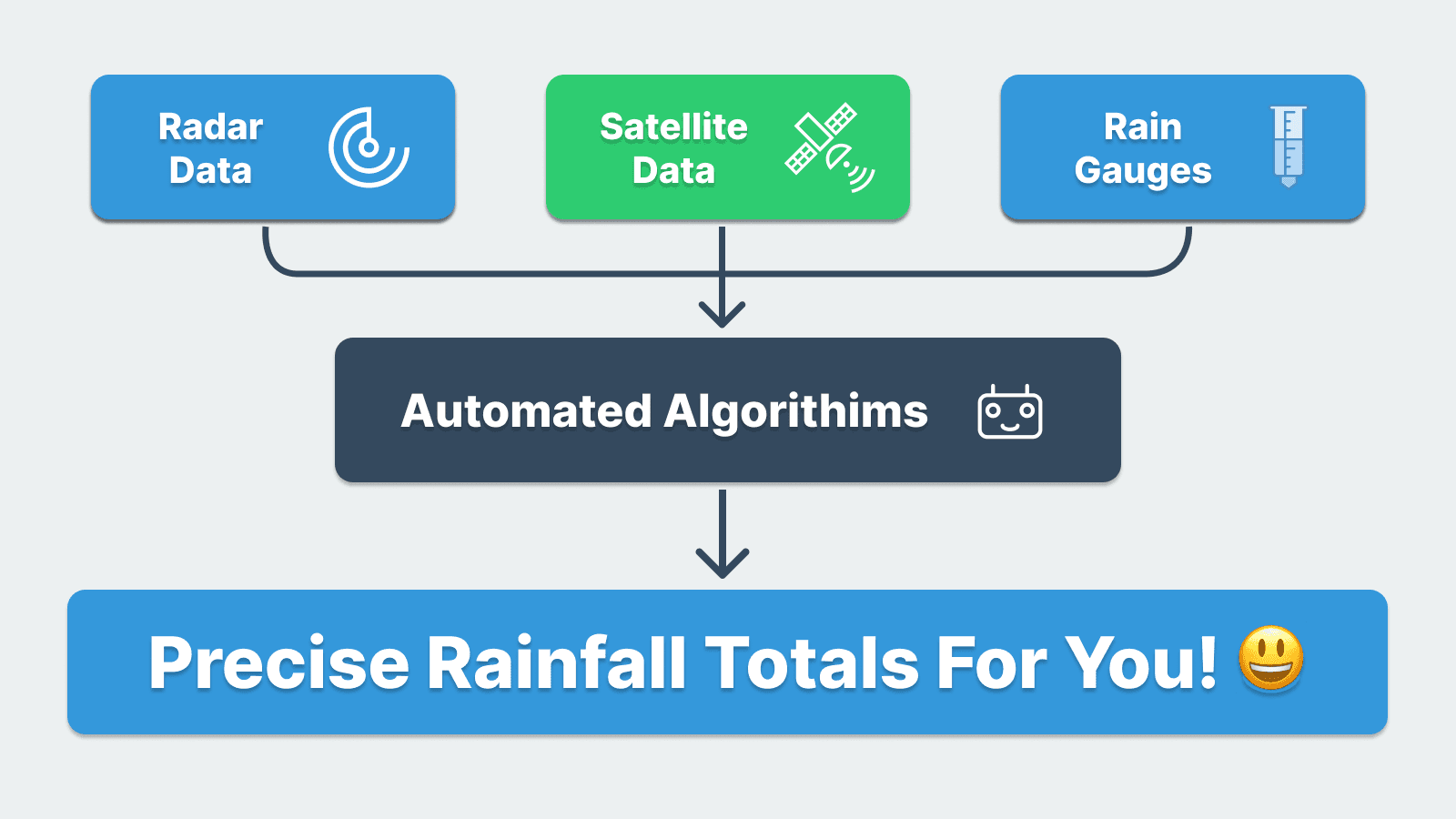
How it Works
We calculate rainfall by processing data from high-resolution radars, ground sensors, and satellite data. This system aggregates inputs from 180 Doppler radars and over 10,000 rain gauges, using scientific models to derive precise rainfall estimates. Our data is hyper-localized with a resolution of 1x1 km, ensuring you receive data tailored exactly to your chosen locations.
Sometimes our data may not match exactly with your physical rain gauge, and here are some reasons why:
Challenges in Matching Physical Rain Gauges
Challenge #1: Rainfall Variability
Rainfall can be highly variable; it can pour 2 inches in one spot while remaining dry just down the road. Our data represents the average rainfall within a 1x1 km area. This means if one part of this area received 2 inches of rain, like your gauge might show, another part could have only gotten 1.25 inches. The average of these readings is why our data might not match exactly with a single rain gauge.
Challenge #2: Wind Speed
Wind speed significantly affects rain gauge measurements. When rain is driven sideways by the wind, physical rain gauges might not capture the full amount of rain. This discrepancy can cause our readings to be higher than those of physical rain gauges. Our data, however, accounts for wind speed by using advanced algorithms that factor in wind direction and intensity from radar and ground sensor data, providing a more accurate rainfall estimate.
Challenge #3: Rain Gauge Types
Different rain gauges can measure rainfall differently due to their design and placement. For instance, a standard rain gauge might capture more rain compared to a tipping bucket rain gauge in the same location, especially during heavy rainfall. This variation in measurements further complicates direct comparisons between our data and individual rain gauge readings.
Challenge #4: Miscellaneous Errors
Rain gauges can experience various errors such as splash in and out, where rain splashes into or out of the gauge, evaporation of collected water, and instrument errors such as freezing, cracking, or complete malfunction. These errors can significantly affect the accuracy of rainfall measurements.
Our System's Challenges
Data Limitations
Our primary data sources are radars strategically placed across the United States, providing overlapping coverage. In most areas of the US, the radar signature is strong and reliable. However, there are regions where radar data may be weak or insufficient. In these cases, we can only rely on rain gauges and satellite data to estimate the rainfall. While satellite data is constantly improving, it currently has limitations in high resolution that can affect accuracy in small areas.
The Future
Despite these challenges, we are continuously working to enhance the precision and accuracy of our rainfall data. With advancements in technology and data processing, we will continue to provide the most accurate and detailed rainfall information possible. Over time, as we collect more data and improve our machine learning algorithms, our measurements will become increasingly accurate for all areas.